Your session is about to expire
Clinical Data Management Process Flow Chart
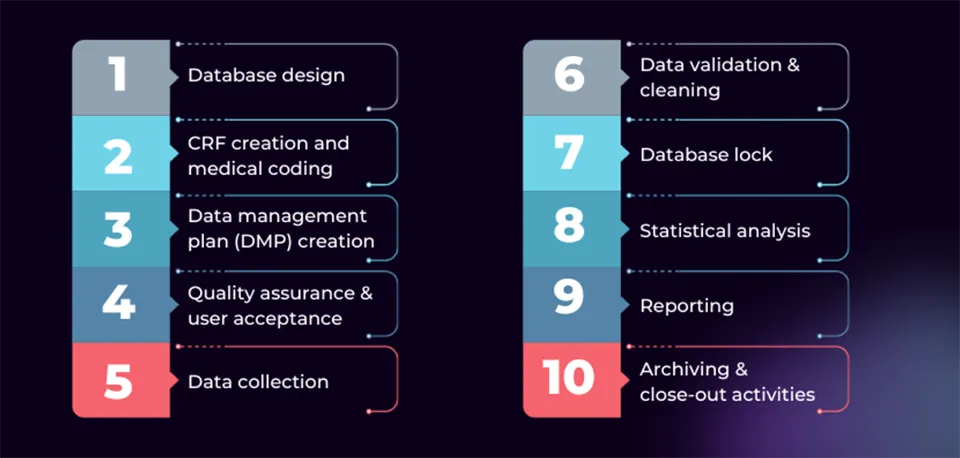
Other Trials to Consider
Popular Categories
Popular Guides
Learn More About These Treatments
Unbiased ResultsWe believe in providing patients with all the options.
Your Data Stays Your DataWe only share your information with the clinical trials you're trying to access.
Verified Trials OnlyAll of our trials are run by licensed doctors, researchers, and healthcare companies.
Back to top
Conditions
Psychology Related
Treatments